Though used extensively in consumer credit and collections, as well as by the financial services community, credit scoring has been slow to catch on in the trade credit environment. However, driven by demands for both greater productivity and greater risk monitoring, credit scoring is now showing signs of gaining momentum.
As you become more familiar with the potential of credit scoring, you'll find that it is not just about the customer approval process. In fact, credit scoring can be used to:
- prioritize collections and assign appropriate collection strategies,
- identify accounts receivable (A/R) portfolio segments that are either over-exposed or under-exposed to risk, and
- save time and money that can then be marshaled into addressing critical issues and pumping more value from your A/R.
Credit scoring, nonetheless, is only as good as both the models you employ and how well you employ those models. It is therefore critical that the implementation of any credit scoring solution be done right.
"Credit scoring, nonetheless, is only as good as both the models you employ and how well you employ those models. It is therefore critical that the implementation of any credit scoring solution be done right"
If your solution is not well thought out, you will not realize the full benefits a successful scoring solution can deliver. The following five key principles will help you make credit scoring a successful endeavor for your organization.
1. Formulate Clearly Defined Business Objectives
The underlying purpose of a credit-scoring model is to predict bad events such as bankruptcy or severe delinquency (e.g., over 90 days past due). You must therefore match the definition of a "bad event" to your business objectives:
- If you want to reduce bad-debt write-offs, you will want to predict defaults.
- If you want to reduce DSO, you may want to focus on reducing the number of customers who pay slower than a certain number of days.
- If you want to maximize collections, say for an A/R portfolio you are acquiring, you may want to identify those accounts that will be the easiest to collect.
- If fraud is a problem, you will want to differentiate between legitimate and fraudulent customers.
The idea is to use credit-scoring models that fit your customer base, credit processes, and objectives. The smaller your customer portfolio, the better you will be served by using a generic score from a commercial credit bureau. Smaller portfolios often will not contain enough "bad events" to generate a valid model.
In addition, if your sample size is too small and too diverse, it will not contain enough similar types of accounts to support a statistical model.
Conversely, the bigger your customer base, the better you will be served by using custom scores. Larger customer bases are more likely to provide a sufficient sample size so that scores can be developed for specific A/R segments.
Moreover, the use of internal data with commercial bureau data often provides more "lift" to your score. Lift describes the increase in good decisions a scoring model can provide for any particular score it generates compared to your former decision process.
Fundamentally, credit scores should turn the odds in your favor. If your customers are mostly large companies that place large orders, credit scoring may be of only limited benefit. However, the higher your volume of small transactions, the more useful credit scores will probably be.
Besides statistically generating more good decisions, you will save a tremendous amount of analyst time, reduce your credit investigation costs, generate more consistent decisions, and fuel quicker decisions. There are therefore many strategic benefits to using credit scores that go beyond their relevance to a particular credit decision.
2. Develop Realistic Expectations
Credit scores are not a cure-all. By themselves, scores will not dramatically improve your receivable's performance. In fact, credit scores may not add value to every segment of your receivables portfolio. Instead, your goal should be to generate more good decisions. To do that, you won't necessarily need to score every customer. That is because credit scores are at their best when used to address well-defined strategic issues as opposed to a one-size-fits-all solution.
Credit scores become a powerful tool when their predictive advantages are coupled with process improvements. Automation is, therefore, an integral part of the scoring process, allowing a good percentage of new customers to be acquired and new orders to be processed without manual intervention.
Most companies that turn to credit scoring do not stop performing credit analysis. Rather, scoring allows you to focus on those critical accounts that require more in-depth analysis. Your larger customers and those accounts that, on the basis of their score, are neither a sure approval nor denial fall into this category-in other words the marginal accounts and other exceptions. However, when reviewing these exception accounts, credit scores also allow you to focus on the data that are not addressed in the score, adding efficiency to the manual review process.
Because of this process component, the implementation of scoring requires a team approach, not just credit department impetus. It needs to be coordinated with sales and marketing as well as with other functions that are affected by your firm's order-to-cash process.
As a side note, one indirect advantage of a team approach to scoring is that sales representatives are less likely to question open-order denials when an objective process, rather than an individual credit analyst, is making the determination. This is because credit scores add validity to the credit department's role.
In addition to making scoring a team effort, don't feel that you have to do it all at once. By starting small and applying scoring models to obvious A/R portfolio segments that are certain to produce winners, you will build credibility throughout your organization for more comprehensive solutions that can be implemented later.
For example, you might start by using scores to process new accounts seeking less than $1000 in credit. Once that process change has proven successful, you can expand to other portfolio segments.
3. Utilize the Most Accurate, Timely, and Comprehensive Information Available
There is one thing you absolutely need to do before designing a credit scoring solution: clean up your receivables. Lots of disputes and deductions can cloud the picture, thereby skewing the results of your credit scores. It is essential that your receivables database be accurate and up-to-date.
You will also need 500 to 1000 "bad events" in order to develop a useful model. If you want to reduce bad-debt write-offs, but don't have that many defaults, you might need to use a commercial score. Or, you might consider the reduction of past-due balances as an alternative objective for scoring. With this objective, you'll probably have enough "bad events" to develop a helpful model.
Make sure you save some bad events for your "validation sample" as well. A validation sample is separate data from the portion of your customer base that you are testing your credit-scoring model against. Its purpose is to allow you to test the results of your scoring model against what happened when you checked credit manually. If you put all your bad events in the model sample, what are you going to validate the model against? You will not get to see how well the model works if you check it against a sample that only contains good outcomes.
Most importantly, it is absolutely vital that you "validate your outcomes" Too many companies are using credit scorecards of their own creation that have not been validated. On occasion, such knowledge-based scorecards have been known to generate results with less predictable results than a random selection of accounts. Even if you purchase a commercial credit score, you need to validate the results that are derived from your portfolio. You may find you need to shop around for a commercial score that better fits your portfolio's characteristics.
4. Use Multiple Measures of Performance to Increase Predictability
Predictability increases with the more characteristics you measure. Payment history is typically the best predictor of future performance. However, by combining payment history with evidence of suits and liens, you will typically get a much more predictive result. Chances are, if you also factor in such things as years in business, company size and financial ratios, you will get even more lift from your score. That is why, if you are building a custom scoring model, you should use as broad a database as possible. You never know what data elements or combinations of data elements will be the most predictive.
5. Continually Monitor Your Scores' Performance and Periodically Revalidate Your Models
Because accounts receivable portfolios are dynamic entities, any credit scoring model you use will deteriorate over time. Scores, therefore, require monitoring and subsequent modification. Furthermore, any scoring solution you implement should be tied to appropriate reporting and analytic capabilities. It is a continuous loop: validate, modify, and then automate.
Not surprisingly, over time, companies using scoring solutions tend to multiply the number of credit scoring models they employ. This phenomenon has everything to do with focus. Scores are at their best when applied to specific situations for specific types of customers.
As you learn to use scores, it is only natural that you will uncover opportunities for more focused applications of scoring solutions to increase accuracy and productivity. In other words, once you take the plunge and start employing credit-scoring models in your order-to-cash process, you are going to find ways to further improve performance through the additional use of scores in new and innovative ways. So don't be afraid to take a stab at scoring. Once you do, you'll be hooked.
Some Key Concepts
Generic scores - A score developed when there is insufficient data to build a proper scorecard. It will typically be based on your experience in your market. It is sometimes called a generic algorithm or GA, or a start-up scorecard. (Thanks to D&B's RAM)
Custom score - Statistical models are often described as a scorecard, a pooled scorecard, and a custom scorecard. A scorecard uses data from one firm. A pooled scorecard uses data from many firms. A custom scorecard blends a statistical model with some of the factors used in a judgmental model. (Thanks to John Sargent of CMS)
Lift - Something that raises, or "lifts" the predictive capability of your model.
Validation Sample - A small percentage of your sample (typically 20 percent) is excluded from your model development process so that it can be used to test the final scorecard. Also known as a Hold-out Sample.
The Five Keys to Ensure Success in Credit Scoring
- Formulate clearly defined business objectives.
- Develop realistic expectations.
- Utilize the most accurate, timely, and comprehensive information available.
- Use multiple measures of performance to increase predictability.
- Continually monitor your scores' performance and periodically revalidate your models.
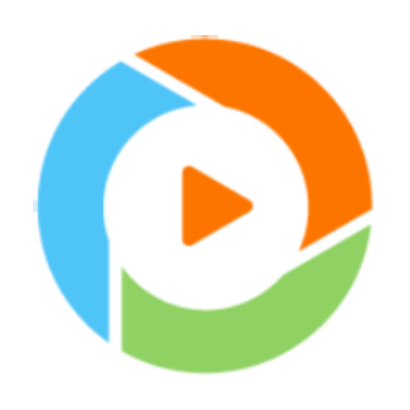